
Stop Guessing, Start Proving: The Role of Data in Refining Your Startup's Problem Hypothesis
What is a Problem Hypothesis?
A problem hypothesis is a key starting point for any startup’s journey. It’s an educated guess about the specific problem your target customer is facing—but it’s just that, a guess. In the early stages of product development, founders often conflate the problem they believe exists with the solution they want to build. This leads to a critical mistake: defining a problem hypothesis that already includes assumptions about the solution. A true problem hypothesis, however, should contain no mention of the solution. It must be focused solely on the problem itself and the context in which it occurs.
Your problem hypothesis acts as the foundation for everything your startup will do. From product features to marketing strategies, everything is built upon this understanding of the customer’s problem. If you include assumptions about the solution at this stage, you risk blurring the line between problem and product, leading to a product that doesn’t solve the right problem or one that doesn’t solve it in the right way.
For example, if your hypothesis is that “small business owners need better accounting software to save time,” you’re already inserting a solution—accounting software—into your thinking. The true problem might be broader, like managing cash flow or improving financial clarity. By focusing too early on your perceived solution, you might miss the deeper pain points your customers are experiencing. A better problem hypothesis might focus on understanding why managing finances is difficult and what the root causes of that struggle are for your customer.

A well-constructed problem hypothesis should answer a few key questions:
- Who is experiencing the problem?
Understand who your target customer is at an individual level, not just an organizational one. Get specific about their role and responsibilities.
- What is the problem they are experiencing?
Define the problem from their perspective, not yours. This is about articulating their pain points, not what you think the problem is.
- What is the negative impact of this problem?
Clarify how this problem affects their day-to-day life or work. Understanding the impact will guide your solution’s value proposition.
- What is the root cause of the problem?
Identify the deeper reasons the problem exists. This systemic understanding helps ensure your solution addresses the real problem, not just symptoms.
- How frequent or urgent is this problem?
Understand how often the problem arises and how pressing it is for your customer. This gives you insights into how motivated they will be to adopt a solution.
- What outcome are they trying to make?
Explore what outcomes they can achieve if the problem we solved.
By focusing entirely on the problem and leaving solutions aside, you create a clear foundation for testing your assumptions. The problem hypothesis is a tool for learning, not proving yourself right. Each iteration and every piece of data gathered during customer discovery helps refine and validate this hypothesis, guiding you toward a solution that addresses a real, validated need in the market.
Use this Custom GPT to create your Startup's Problem Hypothesis Now
Why Data is Essential for Validating Assumptions
At the core of any startup’s success is its ability to test assumptions, and the most crucial assumption of all is the problem hypothesis. Founders often make educated guesses about what their target customers need, but guesses, no matter how informed, aren’t enough. That’s where data comes in. Without it, you’re simply building on speculation, which can lead to significant missteps.
Data plays a critical role in both validating and invalidating assumptions. Validation is straightforward: you gather evidence from customers that supports your hypothesis. For example, through customer interviews, you might confirm that managing cash flow is indeed a significant pain point for small business owners. However, data is equally important for invalidation, which is often overlooked. Invalidation reveals that your hypothesis might be wrong or incomplete, allowing you to course-correct before wasting valuable resources on building a solution for a non-existent or low-priority problem. Founders need to adopt a growth mindset where proving a hypothesis wrong is not a failure—it’s progress toward a better understanding of the customer.
That said, it’s important to recognize that not everything can be measured. While data provides an essential foundation, founders should still rely on their intuition and creativity when interpreting it. Intuition often drives innovative ideas, but those ideas must be tested against real-world data to ensure they address an actual market need. Founders must find a balance between gut feelings and hard evidence, recognizing that data refines intuition, not replaces it.
A key reason startups fail is not because the idea was inherently bad, but because they didn’t properly assess the risks. Data helps mitigate those risks by revealing what the real problems are and how significant they are to the customer. For instance, a founder might assume their market is desperate for an all-in-one inventory management tool, but customer feedback might indicate that what’s truly needed is a simpler integration with existing software rather than an entirely new tool. Without data, that critical distinction could be missed, leading the startup down the wrong path.
By actively collecting data, startups can focus their efforts on solving problems that customers truly care about. This doesn’t stifle innovation; rather, it ensures that innovative ideas are grounded in real customer needs. Founders often fear that data collection will slow them down, but it’s quite the opposite—data speeds up progress by reducing the likelihood of developing the wrong solution.
Data serves two main functions in the validation process:
- Validation of Assumptions:
Qualitative and quantitative data from customer interviews, surveys, and user behavior help confirm that your hypothesis is correct. This feedback gives you the confidence that you’re addressing a real, painful problem.
- Invalidation of Assumptions:
Equally important is discovering that a hypothesis is incorrect or only partially right. Invalidating an assumption early on saves you from spending time and resources building a solution that no one needs.
Founders who rely solely on assumptions without data fall into the trap of confirmation bias, where they only see what supports their beliefs. In contrast, data forces you to confront the reality of your market and adjust accordingly. By gathering objective evidence to validate or invalidate assumptions, startups can refine their problem hypothesis and increase their chances of building a product that solves a real, urgent problem.
How Continuous Customer Discovery Keeps Your Hypothesis Aligned with the Market
Customer discovery is not a one-time activity—it's a continuous process that keeps your startup aligned with the evolving needs of your market. Many founders make the mistake of treating discovery as a box to check early in the development cycle, but in reality, markets shift, customer needs change, and new problems emerge over time. The only way to ensure your problem hypothesis stays relevant is by consistently engaging with your target audience through ongoing discovery.
This continuous feedback loop ensures that you’re always testing and refining your assumptions about the problem. Early insights might give you a good starting point, but ongoing customer discovery reveals new insights and unexpected nuances that can significantly impact your understanding of the customer’s problem. A problem that seemed like the core issue at first may turn out to be a symptom of a deeper, more systemic problem—one that’s more urgent for the customer to solve.
Why Continuous Discovery Matters
Startups that adopt continuous customer discovery are better positioned to adapt to shifts in the market. Customer needs can evolve as new technologies emerge, competitors enter the space, or industries change. By staying in constant contact with your customers, you can spot these shifts early and adjust your problem hypothesis—and ultimately your product—before you invest too heavily in the wrong direction.
For example, a founder may initially hypothesize that small business owners need a tool to manage invoices, only to discover through continuous discovery that automation of their entire billing process is a bigger pain point. By regularly engaging with customers, you can spot these trends early and refine your hypothesis accordingly, ensuring that your solution remains valuable as the market changes.

Testing and Retesting Assumptions
Every startup begins with assumptions, but assumptions need to be tested continuously to stay accurate. Even after an initial problem hypothesis has been validated, it’s essential to keep testing those assumptions as new data comes in. What was true about your customers six months ago might not be true today.
Ongoing discovery ensures that you’re not just building based on outdated assumptions. By continuously gathering new data, you avoid the trap of confirmation bias, where founders see only the evidence that supports their initial beliefs and ignore conflicting data. Continuous discovery pushes you to test and re-test, keeping you flexible and open to pivoting when necessary.
Identifying New Opportunities and Managing Risks
One of the biggest benefits of continuous discovery is that it allows you to uncover emerging opportunities or identify risks before they become critical. As you gather more insights from customers, you may find that new problems have surfaced, or that adjacent markets could benefit from a similar solution. This helps your startup stay agile and ready to explore new avenues of growth.
Likewise, continuous discovery allows you to spot potential risks. You can detect early warning signs that your initial hypothesis may be off-track or that competitors are solving the problem in a more effective way. By managing these risks proactively, you reduce the chances of building a product that becomes irrelevant.
Iterating with Real-Time Feedback
The insights gathered from continuous discovery feed directly into your product development process, enabling real-time iteration. Instead of waiting until after launch to discover whether your solution works, you’re constantly adjusting and improving your hypothesis and product based on fresh feedback. This keeps your solution aligned with real customer needs, increasing the likelihood of building something that resonates with the market.
In short, continuous customer discovery is the engine that drives adaptability. It helps you avoid stagnation, refine your problem hypothesis as the market evolves, and ensure that your product is always grounded in real, current customer needs. By maintaining this ongoing feedback loop, you maximize your chances of success in a dynamic and competitive landscape.
The Role of Iterative Refinement
Iterative refinement is the process of continuously improving your problem hypothesis and product through cycles of feedback, learning, and adaptation. In a startup environment, assumptions about your customer’s problems and needs are rarely correct from the outset. Through iterative refinement, founders can progressively evolve their understanding of the customer’s pain points and adjust their product development to ensure they’re solving the right problem.
The goal of iterative refinement is not to confirm that your initial assumptions were right, but to use data and insights to make incremental improvements to your problem hypothesis. Each new piece of information, whether from customer interviews, surveys, or product usage data, challenges your existing assumptions. This ongoing process of testing, learning, and adjusting is what separates successful startups from those that cling too tightly to their original ideas.
How Iterative Refinement Works
- Gather Data:
Start by collecting qualitative and quantitative data from your customers through continuous discovery efforts. Customer interviews, behavioral analytics, and usage metrics help identify whether your problem hypothesis holds true or needs adjustment.
- Analyze Patterns:
As you gather data, look for patterns or recurring themes in customer feedback. These patterns help you determine if your assumptions are valid or if new insights reveal deeper, more important issues to address.
- Refine the Hypothesis:
Based on what you’ve learned, adjust your problem hypothesis. You may find that the problem you initially identified is only part of a broader challenge your customers face, or that an entirely different issue is more pressing. Refine your hypothesis to reflect this updated understanding.
- Test Again:
Once the hypothesis has been refined, continue testing it through customer discovery and feedback. This cycle of testing and refinement should be continuous to ensure that your product development stays aligned with evolving customer needs.
Why Iterative Refinement is Essential
The iterative refinement process is critical because it allows startups to make small adjustments rather than large, disruptive pivots. By incorporating feedback early and often, you avoid the risk of building a product based on outdated or incorrect assumptions. This process ensures that your understanding of the problem grows deeper and more accurate over time.
Founders often fall into the trap of thinking they’ve discovered the right problem to solve and then focus solely on building the solution. But customer needs change, and the market evolves. Without iterative refinement, there’s a risk of investing too much time and money in a solution that no longer fits the market’s demands. By staying flexible and regularly refining your hypothesis, you ensure that you’re always on track to solving the right problem.
Iterating for Better Products
Iterative refinement doesn’t just benefit the problem hypothesis; it directly impacts the product itself. As you refine your understanding of the problem, you can better prioritize product features, messaging, and user experience. This allows you to deliver a solution that truly addresses your customers’ pain points.
For example, if you discover through customer feedback that a particular feature isn’t solving the intended problem, you can make quick adjustments rather than waiting for a full product release. By continuously refining both your hypothesis and product, you’re able to iterate more quickly and efficiently, leading to a product that aligns closely with real customer needs.
Avoiding Stagnation
Finally, iterative refinement helps startups avoid stagnation. Markets shift, competitors evolve, and customer expectations change. By consistently gathering data and refining your hypothesis, you ensure that your startup remains agile and responsive to these changes. This agility is what keeps successful startups ahead of the curve.
In short, iterative refinement is about staying flexible and responsive. It allows you to adjust to new insights, refine your understanding of the problem, and build a product that evolves alongside your customers’ needs.
Use this Custom GPT to create your Startup's Problem Hypothesis Now
Consequences of Not Collecting Data
Building a startup without actively collecting data to validate or invalidate your assumptions is like navigating uncharted waters without a map. You might set off with a clear vision of where you’re headed, but without data to guide you, you’re likely to get lost. Startups that don’t prioritize data collection often fall into common traps—building solutions for the wrong problems, wasting valuable resources, and missing critical opportunities. Understanding these risks can save your startup from the pitfalls that lead to failure.
Building the Wrong Solution
One of the most significant risks of skipping data collection is building a product that doesn’t address a real or urgent problem. When founders don’t engage in customer discovery or gather enough data to validate their problem hypothesis, they often create solutions based on their own assumptions rather than their customers’ actual needs.
This leads to a situation where the product might be well-designed and feature-rich but fails to resonate with the market because it doesn’t solve a meaningful problem. Customers either won’t see the value or won’t be willing to pay for the solution because it doesn’t address their most pressing pain points. For example, a company might spend months building a complex project management tool, only to find out through post-launch feedback that customers primarily needed a simpler way to collaborate, not all the extra features.
Wasting Time and Resources
Another consequence of neglecting data collection is the waste of resources—both time and money. Developing and launching a product based on untested assumptions can lead to costly pivots or, worse, a complete failure to find product-market fit. When founders fail to validate their assumptions early, they risk investing in features, marketing strategies, and technologies that don’t align with what their target customers actually need.
By contrast, startups that collect data continuously and iterate based on customer feedback are able to allocate their resources more effectively. They build what matters most, minimizing the chances of having to go back and fix or overhaul their product after it’s already been launched.
Missed Opportunities
Startups that don’t actively gather data also risk missing out on valuable insights and opportunities. Customer discovery and ongoing feedback loops don’t just help validate existing assumptions—they often reveal new problems, adjacent market opportunities, or additional customer segments that founders may not have considered. When startups fail to engage deeply with their market, they limit their ability to discover these insights and miss opportunities to differentiate or expand their product offering.
Confirmation Bias and Inflexibility
Without a structured approach to collecting data, founders often fall into the trap of confirmation bias—the tendency to look for evidence that supports their pre-existing beliefs while ignoring contradictory information. This bias leads to stubbornly sticking to a flawed problem hypothesis and missing signals from the market that something is off.
A product built on confirmation bias risks becoming irrelevant. In contrast, data forces founders to confront the reality of their market. It helps them make data-driven decisions rather than relying on gut feelings or personal biases. This flexibility to change course based on real-world feedback is crucial for a startup’s long-term success.

Real-World Examples
The consequences of ignoring data are illustrated by companies like Juicero and Quibi, which invested millions into building products based on untested assumptions. Both failed because they didn’t validate whether the market truly needed what they were offering, leading to costly mistakes and, eventually, shutdown.
Data as a Safeguard
Failing to collect data means flying blind. Without data, you risk building the wrong solution, wasting resources, and missing out on critical market opportunities. By continuously gathering data to test your assumptions, you can safeguard your startup from these common pitfalls and set yourself up for success in a competitive market.
Best Practices for Gathering Data
Effective data collection is the backbone of validating or invalidating your problem hypothesis, but it’s not just about gathering data for the sake of it—it’s about gathering the right data in a way that informs decision-making. Whether through customer interviews, surveys, or behavioral analytics, the data you collect should provide actionable insights that help refine your problem hypothesis and guide product development. Here are some best practices to ensure your data collection is strategic and meaningful.
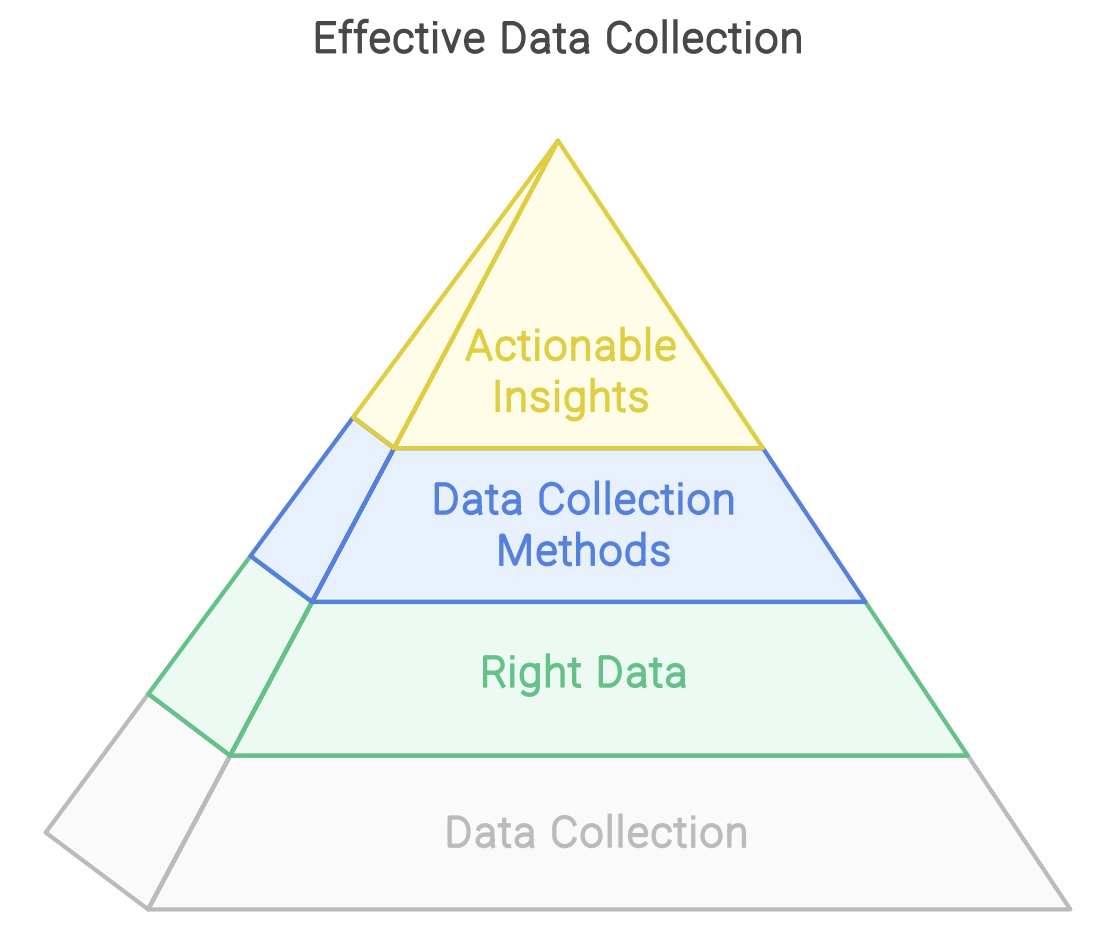
1. Structured Customer Interviews (Qualitative Data)
Customer interviews are one of the most powerful tools for gathering deep, qualitative insights. These conversations allow you to uncover the motivations, frustrations, and behaviors that drive your target customers.
- Ask Open-Ended Questions:
Instead of asking yes/no questions, focus on open-ended questions like “Can you walk me through how you handle this issue?” This encourages customers to share their experience in detail, providing insights you may not have considered.
- Don’t Lead the Customer:
Avoid framing questions that steer customers toward your desired outcome. For example, asking “Do you think this tool would save you time?” leads the customer toward agreeing with you. Instead, ask “What’s the most frustrating part of this process for you?” to uncover real pain points.
- Listen More, Talk Less:
The goal of these interviews is to learn from your customers, not to sell your product. Keep the focus on their experiences and resist the urge to pitch or guide the conversation toward your solution.
2. Surveys and Polls for Quantitative Validation
Surveys are a great way to gather quantitative data from a larger audience, providing insights into how widespread a particular problem is and how customers prioritize different challenges.
- Keep Surveys Short and Targeted:
People are more likely to complete shorter surveys. Focus on specific questions that give you clear insights, such as “How often does this problem occur?” or “How much time do you spend dealing with this issue each week?”
- Use Rating Scales and Yes/No Questions:
These help quantify the severity or frequency of problems. For example, you might ask, “On a scale of 1 to 10, how much does this issue impact your productivity?”
- Offer Incentives:
To increase response rates, consider offering small incentives such as discounts or access to exclusive content.
3. Behavioral Data (What Customers Do vs. What They Say)
While customer interviews and surveys offer valuable insights, there’s often a gap between what people say they do and what they actually do. This is where behavioral data—information on how customers interact with your product—comes in.
- Use Analytics Tools:
Tools like Google Analytics, Mixpanel, and Hotjar track user behavior on your website or product. This data shows which features customers use the most, where they drop off, and what actions they take, providing insights into real-world usage.
- Run A/B Tests:
A/B testing allows you to compare two versions of a feature or design to see which performs better. It’s a simple way to gather direct data on what works and what doesn’t, ensuring you’re building based on what users respond to best.
4. Establish a Feedback Loop
Data collection shouldn’t be a one-time effort. Establish a feedback loop where you’re continuously gathering and analyzing customer data to keep your product aligned with market needs.
- Integrate Feedback Mechanisms:
Build feedback tools directly into your product—like a feature request button or post-purchase survey—to collect ongoing feedback.
- Regularly Review Insights:
Set up a process to regularly review and discuss customer feedback as a team. Whether monthly or quarterly, this practice helps ensure that customer needs remain front and center as your product evolves.
5. Balance Qualitative and Quantitative Data
Both qualitative and quantitative data are essential, and they complement each other. Use customer interviews and surveys to understand the “why” behind customer behavior, and leverage analytics and behavioral data to validate or refine those insights at scale.
- Use Qualitative Data to Inform Quantitative Questions:
Insights from interviews can help guide the design of your surveys, ensuring you ask the right questions in the right way.
- Look for Patterns:
Look for consistent patterns between your qualitative and quantitative data. If customers repeatedly mention a particular pain point in interviews and survey responses align with that pain, it’s likely a critical issue worth addressing.
The 6-Question Tool for Writing Your Problem Hypothesis
To help early-stage founders craft a clear and actionable problem hypothesis, it’s important to have a structured approach. The 6-question tool provides a simple framework that guides founders through the process of identifying and articulating the problem their target customers are facing—without jumping to solutions prematurely. This tool not only helps clarify the problem but also ensures that the hypothesis is grounded in customer insights and real-world data.
Here’s how you can use the 6-question tool to define your problem hypothesis:
1. Who is the individual experiencing the problem?
This question helps you narrow down exactly who you are solving the problem for. It’s not enough to say “small businesses” or “tech companies”—you need to get specific. Who within those organizations is feeling the pain? Is it the CEO, the marketing manager, or a frontline employee?
The goal here is to understand the individual’s role and responsibilities within the organization. By zeroing in on the person experiencing the problem, you gain deeper insight into how the problem impacts their day-to-day life, and you’ll avoid making overly broad assumptions.
2. What is the problem they are experiencing?
This question pushes founders to articulate the problem from the customer’s perspective, not their own. Often, founders describe the problem as they see it, which may not fully capture how the customer experiences it. Ask yourself: How would the customer describe their frustration or challenge in their own words?
This distinction is critical because your problem hypothesis needs to be rooted in the customer’s reality. What they see as the problem is what matters—not what you think the problem is.
3. What is the negative impact of this problem?
Understanding the negative consequences of the problem gives context to its importance. What’s the cost of this problem to the customer? Does it slow down their productivity? Hurt their bottom line? Increase their stress or workload?
This step helps founders hypothesize what success would look like for the customer. If your solution effectively addresses the problem, what would the customer avoid or achieve? It also helps you prioritize problems that have the most significant impact on your target audience.
4. What is the root cause of the problem?
Identifying the root cause is essential for building an effective solution. Many problems are symptoms of deeper issues, and unless you address the root cause, your solution may only offer a temporary fix.
Consider why the problem exists in the first place. Is it due to outdated processes, poor communication, or technical limitations? Understanding the deeper, systemic reasons behind the problem allows you to design a solution that solves the real issue.
5. How frequent is this problem?
Understanding the frequency of the problem helps you gauge its urgency. Is this a problem that happens daily, weekly, or sporadically?
A problem that occurs frequently is often more urgent to solve, while a problem that happens less often may not have the same priority. Frequency helps founders assess how motivated customers will be to find a solution, which is critical in determining product-market fit.
6. What outcome are they trying to achieve?
Finally, define the desired outcome from the customer’s point of view. What is the end goal they are trying to reach? Is it saving time, improving accuracy, reducing costs, or streamlining a process?
Understanding the desired outcome gives you a clear direction for how your solution should perform. It also helps you measure whether your product is delivering real value.
Use this Custom GPT to create your Startup's Problem Hypothesis Now
Putting It All Together
By answering these six questions, you create a problem hypothesis that is not only clear and actionable but also closely aligned with customer needs. Your hypothesis will no longer be based on assumptions or personal intuition—it will be grounded in insights gathered from actual customer interactions.
This tool also acts as a guidepost for future iterations. As you gather more data and engage in continuous customer discovery, you can revisit these questions to refine your hypothesis, ensuring that it evolves alongside your understanding of the market.
With this structured approach, founders can avoid the common pitfall of building solutions based on vague or misaligned assumptions, and instead focus on addressing the most pressing, clearly defined problems their target customers face.